Image recognition accuracy: An unseen challenge confounding todays AI Massachusetts Institute of Technology

For instance, a dog image needs to be identified as a “dog.” And if there are multiple dogs in one image, they need to be labeled with tags or bounding boxes, depending on the task at hand. The quality and diversity of the training dataset play a crucial role in the model’s performance, and continuous training may be necessary to enhance its accuracy over time and adapt to evolving data patterns. Yes, image recognition models need to be trained to accurately identify and categorize objects within images. Evaluate the specific features offered by each tool, such as facial recognition, object detection, and text extraction, to ensure they align with your project requirements. Lapixa’s AI delivers impressive accuracy in object detection and text recognition, crucial for tasks like content moderation and data extraction.
Unlike traditional methods that focus on absolute performance, this new approach assesses how models perform by contrasting their responses to the easiest and hardest images. The study further explored how image difficulty could be explained and tested for similarity to human visual processing. Using metrics like c-score, prediction depth, and adversarial robustness, the team found that harder images are processed differently by networks.
This produces labeled data, which is the resource that your ML algorithm will use to learn the human-like vision of the world. Naturally, models that allow artificial intelligence image recognition without the labeled data exist, too. They work within unsupervised machine learning, however, there are a lot of limitations to these models. If you want a properly trained image recognition algorithm capable of complex predictions, you need to get help from experts offering image annotation services.
Image recognition with deep learning is a key application of AI vision and is used to power a wide range of real-world use cases today. “One of my biggest takeaways is that we now have another dimension to evaluate models on. We want models that are able to recognize any image even if — perhaps especially if — it’s hard for a human to recognize. The project identified interesting trends in model performance — particularly in relation to scaling. Larger models showed considerable improvement on simpler images but made less progress on more challenging images. You can foun additiona information about ai customer service and artificial intelligence and NLP. The CLIP models, which incorporate both language and vision, stood out as they moved in the direction of more human-like recognition.
Facial Recognition
Other face recognition-related tasks involve face image identification, face recognition, and face verification, which involves vision processing methods to find and match a detected face with images of faces in a database. Deep learning recognition methods are able to identify people in photos or videos even as they age or in challenging illumination situations. This AI vision platform lets you build and operate real-time applications, use neural networks for image recognition tasks, https://chat.openai.com/ and integrate everything with your existing systems. While pre-trained models provide robust algorithms trained on millions of datapoints, there are many reasons why you might want to create a custom model for image recognition. For example, you may have a dataset of images that is very different from the standard datasets that current image recognition models are trained on. In this case, a custom model can be used to better learn the features of your data and improve performance.
- Larger models showed considerable improvement on simpler images but made less progress on more challenging images.
- At about the same time, a Japanese scientist, Kunihiko Fukushima, built a self-organising artificial network of simple and complex cells that could recognise patterns and were unaffected by positional changes.
- While pre-trained models provide robust algorithms trained on millions of datapoints, there are many reasons why you might want to create a custom model for image recognition.
- Through extensive training on datasets, it improves its recognition capabilities, allowing it to identify a wide array of objects, scenes, and features.
- If the data has all been labeled, supervised learning algorithms are used to distinguish between different object categories (a cat versus a dog, for example).
- There are a few steps that are at the backbone of how image recognition systems work.
“It’s visibility into a really granular set of data that you would otherwise not have access to,” Wrona said. A digital image is composed of picture elements, or pixels, which are organized spatially into a 2-dimensional grid or array. Each pixel has a numerical value that corresponds to its light intensity, or gray level, explained Jason Corso, a professor of robotics at the University of Michigan and co-founder of computer vision startup Voxel51. The model is periodically re-evaluated and the entire process from the previous two steps is repeated in the background. While highly effective, the cost may be a concern for small businesses with limited budgets, particularly when dealing with large volumes of images.
With Alexnet, the first team to use deep learning, they managed to reduce the error rate to 15.3%. Trendskout applies different types of feature transformation and extraction, in interaction with the hyper-tuning step. For example, a photo can first be transformed via PCA to a lower dimensional structure, high contrast filters can be applied to it, or certain features can be pre-selected via feature extraction. This step is similar to the data processing applied to data with a lower dimensionality, but uses different techniques. As with classification, annotated data is also often required here, i.e. training data on which the system can learn which patterns, objects or images to recognize. Creating a custom model based on a specific dataset can be a complex task, and requires high-quality data collection and image annotation.
You’re looking to create a new project. What are the best AI-powered image recognition tools to use?
Clarifai’s custom training feature allows users to adapt the software for specific use cases, making it a flexible solution for diverse industries. Clarifai is scalable, catering to the image recognition needs of both small businesses and large enterprises. The software offers predictive image analysis, providing insights into image content and characteristics, which picture recognition ai is valuable for categorization and content recommendations. It can also detect boundaries and outlines of objects, recognizing patterns characteristic of specific elements, such as the shape of leaves on a tree or the texture of a sandy beach. The software easily integrates with various project management and content organization tools, streamlining collaboration.

Efforts began to be directed towards feature-based object recognition, a kind of image recognition. The work of David Lowe “Object Recognition from Local Scale-Invariant Features” was an important indicator of this shift. The paper describes a visual image recognition system that uses features that are immutable from rotation, location and illumination.
For more details on platform-specific implementations, several well-written articles on the internet take you step-by-step through the process of setting up an environment for AI on your machine or on your Colab that you can use. It then combines the feature maps obtained from processing the image at the different aspect ratios to naturally handle objects of varying sizes. The terms image recognition and image detection are often used in place of each other.
Image recognition plays a crucial role in medical imaging analysis, allowing healthcare professionals and clinicians more easily diagnose and monitor certain diseases and conditions. Image and Pattern recognition is one of the available Trendskout AI Flow analysis-functions. It’s crucial to select a tool that not only meets your immediate needs but also provides room for future scalability and integration with other systems. While Lapixa offers API integration, users with minimal coding experience may find implementation and maintenance challenging.
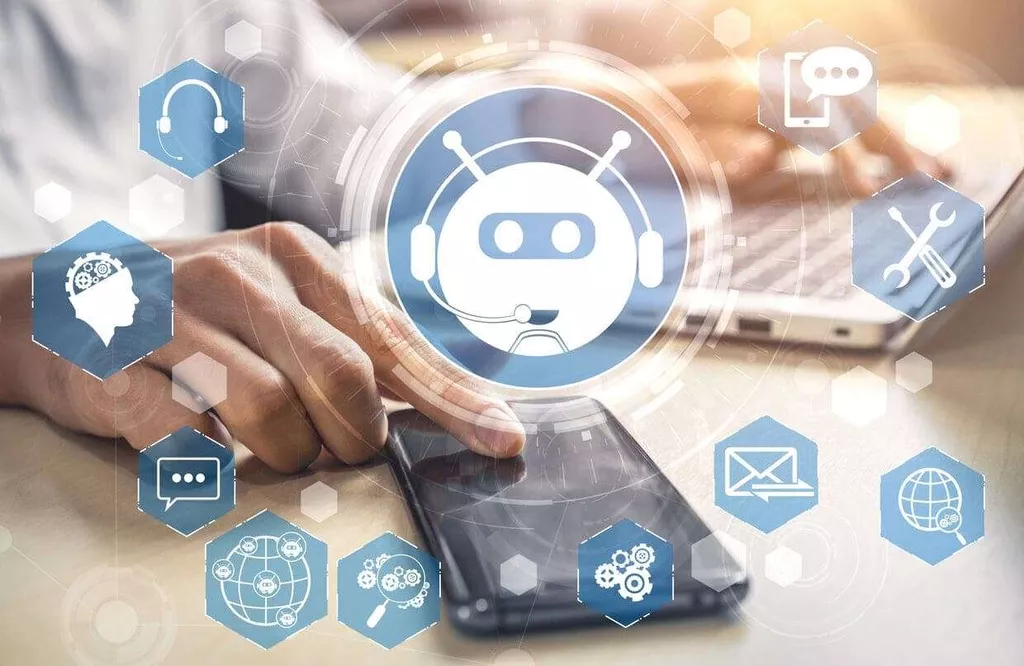
Performance is also essential; you should consider the speed and accuracy of the tool, as well as its computing power and memory requirements. Lastly, you should make sure that the tool integrates well with other tools and platforms, supports multiple formats and sources of images, and works with different operating systems and devices. Computer vision (and, by extension, image recognition) is the go-to AI technology of our decade. MarketsandMarkets research indicates that the image recognition market will grow up to $53 billion in 2025, and it will keep growing.
It can be big in life-saving applications like self-driving cars and diagnostic healthcare. But it also can be small and funny, like in that notorious photo recognition app that lets you identify wines by taking a picture of the label. In many cases, a lot of the technology used today would not even be possible without image recognition and, by extension, computer vision. The CNN then uses what it learned from the first layer to look at slightly larger parts of the image, making note of more complex features. It keeps doing this with each layer, looking at bigger and more meaningful parts of the picture until it decides what the picture is showing based on all the features it has found. Image recognition tools have become integral in our tech-driven world, with applications ranging from facial recognition to content moderation.
Study: Deep neural networks don’t see the world the way we do
Image recognition and pattern recognition are specific subtypes of AI and Deep Learning. This means that a single data point – e.g. a picture or video frame – contains lots of information. The high-dimensional nature of this type of data makes neural networks particularly suited for further processing and analysis – whether you are looking for image classification or object or pattern recognition. Currently, convolutional neural networks (CNNs) such as ResNet and VGG are state-of-the-art neural networks for image recognition. In current computer vision research, Vision Transformers (ViT) have recently been used for Image Recognition tasks and have shown promising results. Image search recognition, or visual search, uses visual features learned from a deep neural network to develop efficient and scalable methods for image retrieval.
Nevertheless, this project was seen by many as the official birth of AI-based computer vision as a scientific discipline. Lawrence Roberts is referred to as the real founder of image recognition or computer vision applications as we know them today. In his 1963 doctoral thesis entitled “Machine perception of three-dimensional solids”Lawrence describes the process of deriving 3D information about objects from 2D photographs. The initial intention of the program he developed was to convert 2D photographs into line drawings. These line drawings would then be used to build 3D representations, leaving out the non-visible lines. In his thesis he described the processes that had to be gone through to convert a 2D structure to a 3D one and how a 3D representation could subsequently be converted to a 2D one.
What Is Image Recognition? – Built In
What Is Image Recognition?.
Posted: Tue, 30 May 2023 07:00:00 GMT [source]
The initial step involves providing Lapixa with a set of labeled photographs describing the items within them. When you feed an image into Azure AI Vision, its artificial intelligence systems work, breaking down the picture pixel by pixel to comprehend its meaning. When you feed a picture into Clarifai, it goes through the process of analysis and understanding. While Imagga provides encryption and authentication features, additional security measures may be necessary to protect sensitive information in collaborative projects. Some people worry about the use of facial recognition, so users need to be careful about privacy and following the rules. It’s powerful, but setting it up and figuring out all its features might take some time.
Agricultural machine learning image recognition systems use novel techniques that have been trained to detect the type of animal and its actions. Image recognition with machine learning, on the other hand, uses algorithms to learn hidden knowledge from a dataset of good and bad samples (see supervised vs. unsupervised learning). The most popular machine learning method is deep learning, where multiple hidden layers of a neural network are used in a model. This usually requires a connection with the camera platform that is used to create the (real time) video images. This can be done via the live camera input feature that can connect to various video platforms via API.
Image recognition is used in security systems for surveillance and monitoring purposes. It can detect and track objects, people or suspicious activity in real-time, enhancing security measures in public spaces, corporate buildings and airports in an effort to prevent incidents from happening. These software systems can identify and categorize objects, scenes, patterns, text, and even activities within digital visual data. Azure AI Vision employs cutting-edge AI algorithms for in-depth image analysis, recognizing objects, text, and providing descriptions of visual content. Like any image recognition software, users should be mindful of data privacy and compliance with regulations when working with sensitive content. The software boasts high accuracy in image recognition, especially with custom-trained models, ensuring reliable results for various applications.
Image recognition tools refer to software systems or applications that employ machine learning and computer vision methods to recognize and categorize objects, patterns, text, and actions within digital images. In some cases, you don’t want to assign categories or labels to images only, but want to detect objects. The main difference is that through detection, you can get the position of the object (bounding box), and you can detect multiple objects of the same type on an image. Therefore, your training data requires bounding boxes to mark the objects to be detected, but our sophisticated GUI can make this task a breeze. From a machine learning perspective, object detection is much more difficult than classification/labeling, but it depends on us.
For industry-specific use cases, developers can automatically train custom vision models with their own data. These models can be used to detect visual anomalies in manufacturing, organize digital media assets, and tag items in images to count products or shipments. Furthermore, image recognition can help you create art and entertainment with style transfer or generative adversarial networks. Additionally, it can be used to gain a better understanding of AI concepts and techniques such as deep learning, neural networks, convolutional layers, and transfer learning. Image recognition is the ability of computers to identify and classify specific objects, places, people, text and actions within digital images and videos. In past years, machine learning, in particular deep learning technology, has achieved big successes in many computer vision and image understanding tasks.

The image is first converted into tiny squares called pixels, considering the color, location, and intensity of each pixel to create a digital format. Imagga significantly boosts content management efficiency in collaborative projects by automating image tagging and organization. It can recognize specific patterns and deduce boundaries and shapes, such as the wing of a bird or the texture of a beach. One of Imagga’s strengths is feature extraction, where it identifies visual details like shapes, textures, and colors.
While early methods required enormous amounts of training data, newer deep learning methods only needed tens of learning samples. This led to the development of a new metric, the “minimum viewing time” (MVT), which quantifies the difficulty of recognizing an image based on how long a person needs to view it before making a correct identification. Once all the training data has been annotated, the deep learning model can be built.
Image recognition is a subset of computer vision, which is a broader field of artificial intelligence that trains computers to see, interpret and understand visual information from images or videos. Imagga is a powerful image recognition tool that uses advanced technologies to analyze and understand the content within images. This process involves analyzing and processing the data within an image to identify and detect objects, features, or patterns. With the help of machine vision cameras, these tools can analyze patterns in people, gestures, objects, and locations within images, looking closely at each pixel. Image recognition software or tools generates neural networks using artificial intelligence. Visive’s Image Recognition is driven by AI and can automatically recognize the position, people, objects and actions in the image.
Experience has shown that the human eye is not infallible and external factors such as fatigue can have an impact on the results. These factors, combined with the ever-increasing cost of labour, have made computer vision systems readily available in this sector. At about the same time, a Japanese scientist, Kunihiko Fukushima, built a self-organising artificial network of simple and complex cells that could Chat PG recognise patterns and were unaffected by positional changes. This network, called Neocognitron, consisted of several convolutional layers whose (typically rectangular) receptive fields had weight vectors, better known as filters. These filters slid over input values (such as image pixels), performed calculations and then triggered events that were used as input by subsequent layers of the network.

The enterprise suite provides the popular open-source image recognition software out of the box, with over 60 of the best pre-trained models. It also provides data collection, image labeling, and deployment to edge devices – everything out-of-the-box and with no-code capabilities. In image recognition, the use of Convolutional Neural Networks (CNN) is also called Deep Image Recognition. However, engineering such pipelines requires deep expertise in image processing and computer vision, a lot of development time and testing, with manual parameter tweaking.
If you’re looking for a new project to challenge your skills and creativity, you might want to explore the possibilities of AI-powered image recognition. Image recognition is a branch of computer vision that enables machines to identify and classify objects, faces, emotions, scenes, and more in digital images. With the help of some tools and frameworks, you can build your own image recognition applications and solve real-world problems. In this article, we’ll introduce you to some of the best AI-powered image recognition tools to use for your project. It is a well-known fact that the bulk of human work and time resources are spent on assigning tags and labels to the data.
Users can fine-tune the AI model to meet specific image recognition needs, ensuring flexibility and improved accuracy. MS Azure AI has undergone extensive training on diverse datasets, enabling it to recognize a wide range of objects, scenes, and even text—whether it’s printed or handwritten. Essentially, image recognition relies on algorithms that interpret the content of an image.
As described above, the technology behind image recognition applications has evolved tremendously since the 1960s. Today, deep learning algorithms and convolutional neural networks (convnets) are used for these types of applications. Within the Trendskout AI software platform we abstract from the complex algorithms that lie behind this application and make it possible for non-data scientists to also build state of the art applications with image recognition. In this way, as an AI company, we make the technology accessible to a wider audience such as business users and analysts.
Imagga’s Auto-tagging API is used to automatically tag all photos from the Unsplash website. Providing relevant tags for the photo content is one of the most important and challenging tasks for every photography site offering huge amount of image content. However, if specific models require special labels for your own use cases, please feel free to contact us, we can extend them and adjust them to your actual needs. We can use new knowledge to expand your stock photo database and create a better search experience.
The AI Trend Skout software also makes it possible to set up every step of the process, from labelling to training the model to controlling external systems such as robotics, within a single platform. OCI Vision is an AI service for performing deep-learning–based image analysis at scale. With prebuilt models available out of the box, developers can easily build image recognition and text recognition into their applications without machine learning (ML) expertise.
Logo detection and brand visibility tracking in still photo camera photos or security lenses. In the area of Computer Vision, terms such as Segmentation, Classification, Recognition, and Object Detection are often used interchangeably, and the different tasks overlap. While this is mostly unproblematic, things get confusing if your workflow requires you to perform a particular task specifically. A native iOS and Android app that connects neighbours and helps local businesses to grow within local communities.
The most popular deep learning models, such as YOLO, SSD, and RCNN use convolution layers to parse a digital image or photo. During training, each layer of convolution acts like a filter that learns to recognize some aspect of the image before it is passed on to the next. A distinction is made between a data set to Model training and the data that will have to be processed live when the model is placed in production. As training data, you can choose to upload video or photo files in various formats (AVI, MP4, JPEG,…).
We usually start by determining the project’s technical requirements in order to build the action plan and outline the required technologies and engineers to deliver the solution. Receive a personalised project estimate and take the first step towards bringing your idea to life. Used for automated detection of damage and assessment of its severity, used by insurance or rental companies. Image recognition benefits the retail industry in a variety of ways, particularly when it comes to task management.